The arena of Artificial Intelligence (AI) is dynamic and continually evolving, opening new frontiers for technology and influencing a multitude of industries worldwide. In focus here is a powerful subset of AI – Generative AI – akin to the most ingenious of artists, conjuring creations from a blank canvas.
The journey starts with understanding the fundamentals of AI, its varying types, and the pivotal role it plays in today’s world. Delving further, we explore the core characteristics that make generative AI stand apart from the rest, and delve into the mechanism and functionality behind it.
To truly grasp its capabilities, a detailed look at the specific algorithms and models such as Generative Adversarial Networks (GANs) and Variational Autoencoders (VAEs) that fuel generative AI is essential. The concepts might seem daunting, but rest assured, they are fascinating.
Understanding the Basics of Artificial Intelligence (AI)
Understanding Artificial Intelligence (AI)
Artificial Intelligence, often referred to as AI, is a broad field of computer science that aims to mimic human intelligence with machine technology. This can involve anything from voice recognition, to decision-making algorithms, to image recognition, and much more. The ultimate goal is for these systems to be able to perform tasks traditionally requiring human intelligence in a more efficient, effective, and accurate way.
Types of Artificial Intelligence
There are two fundamental groups or types of AI: Narrow AI, which is a system designed to perform a single task such as voice recognition, and General AI, which is a system designed with the ability to perform any cognitive function that a human can do. Most AI systems that we see today, like the voice assistants Siri or Alexa, are examples of Narrow AI.
The Importance of AI
The importance of AI cannot be understated. Its uses are vast, as it can be applied across a multitude of industries such as healthcare, finance, transport, and many others. AI can perform tasks faster than a human can, make predictions based on large datasets, and work without fatigue. More importantly, as it continues to advance, AI is expected to drive transformational change in the way we live and work.
Machine Learning and Deep Learning
Machine Learning (ML) is a subset of AI that involves the science of getting computers to learn and act similarly to humans by feeding them data and information in the form of observations and real-world interactions. Deep Learning, on the other hand, is a subset of ML that focuses on algorithms inspired by the structure and function of the human brain called artificial neural networks.
Generative AI: Its Mechanism and Significance
Generative AI belongs to the field of machine learning where AI algorithms create new data instances that resemble your training data. For instance, GANs (Generative Adversarial Networks) are a type of AI algorithm that has two parts: a generator that creates new instances and a discriminator that tries to differentiate between real and fake instances. The two parts work together, effectively refining the output.
Generative AI is gaining significance as it evolves beyond merely performing tasks to becoming a creator and pioneer. The ability to ‘generate’ and ‘create’ was thought of as an exclusively human trait, but Generative AI challenges that belief, sharing in this unique sphere. This development brings a revolution on the horizon that is bound to massively alter the way we live, work, and inspire creations.
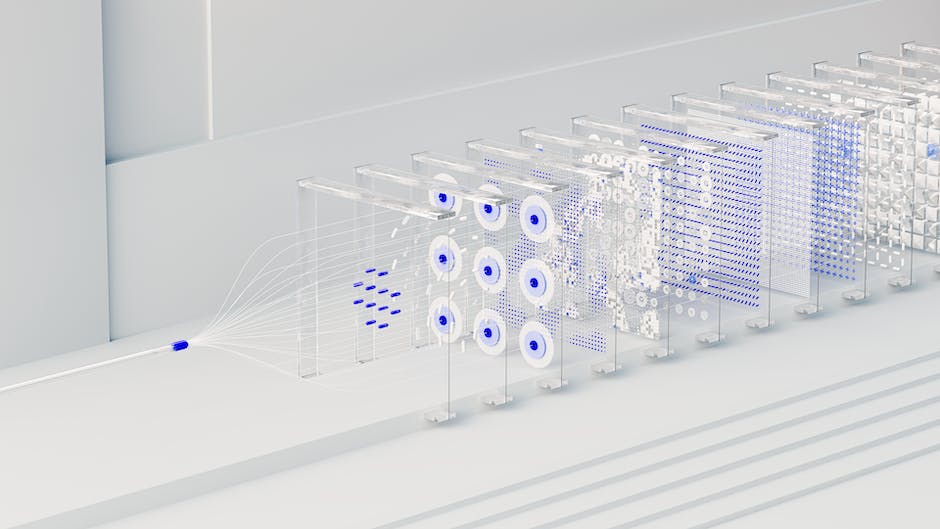
A Deep Dive into Generative AI: How It Works and Why It Matters?
Getting to Know Generative AI
Often referred to as generative adversarial networks (GANs), Generative AI is a subset of artificial intelligence that employs machine learning algorithms to generate data mirroring that which was originally given to it. This type of AI differs from others by virtue of its capabilities, instead of making predictions or decisions based on provided data, generative AI produces new data that closely resembles the original input.
How Generative AI Works
The basic principle of generative AI revolves around two neural networks – the Generator and the Discriminator – performing in a competition with each other. The Generator creates a new piece of data, such as a human image, while the Discriminator assesses the generated data. If the Discriminator finds the generated data to be fake or dissimilar to the original data, it sends feedback to the Generator. Using this feedback, the Generator then refines its data creation process. This iterative process continues until the Discriminator can no longer differentiate the generated data from the actual data.
Characteristics of Generative AI
Generative AI primarily focuses on generating data that can be both understandable and valuable to humans. This data can be in any form, including text, audio, or visual. The output should be creative but should also retain the core characteristics of the source material. The process of data generation and refinement carries on until the data is virtually indistinguishable from human-created content.
Applications of Generative AI
Generative AI technology has found practical applications in various fields. In the art world, it enabled the creation of artworks that were exhibited, sold, and even auctioned at substantial prices. In the field of healthcare, AI-based drug discovery systems generate synthesizable and potentially effective drug candidates. Generative AI is also used in the auto industry for designing car parts. Text generation tools, such as AI-based story or poem generators, exemplify its application in content creation. It also finds use in generating gaming environments and creating modifications to existing video games.
Understanding Generative AI and Its Significance
In the realm of activities traditionally requiring intensive human creativity and input, Generative AI has emerged as a game-changing factor. It has the potential to automate these processes to an impressive level and deliver solutions on an unforeseen scale.
The significance of Generative AI is gaining traction in the face of challenges like managing enormous data volumes and the demand for tailored solutions across different sectors. At the same time, questions are being raised about the ethical and regulatory aspects of the origin, authenticity, and ownership of AI-generated content. Thus, it’s crucial to harness Generative AI in a balanced manner, taking into account both its incredible potential and the significant challenges it presents.
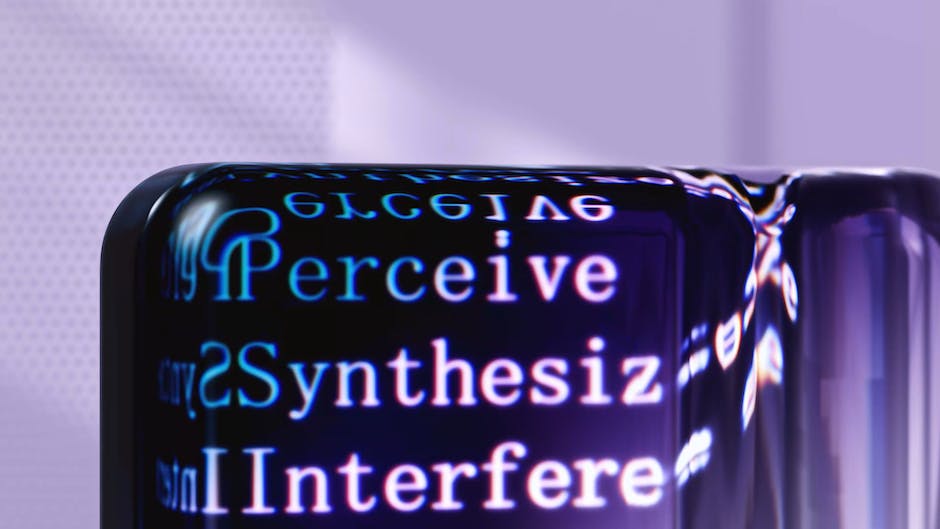
Generative AI Algorithms and Models
An Overview of Generative AI Algorithms and Models
Algorithms and models of Generative AI, such as Generative Adversarial Networks (GANs) and Variational Autoencoders (VAEs), have been developed with the goal of creating new instances of data that resemble the original input. These algorithms have been structured to comprehend the underlying data patterns and reproduce similar data constructs.
Generative Adversarial Networks (GANs)
GANs consist of two primary elements: a generator and a discriminator. The generator creates novel data instances, while the discriminator tries to distinguish between real and fake data. The interaction between these elements is akin to a game of cat and mouse, where the generator constantly strives to trick the discriminator, and the discriminator continually learns to better differentiate the real from the artificially generated data.
One advantage of GANs is their ability to generate high-quality, realistic images or data. They have been widely used in applications such as generating images, enhancing image resolution, and generating photo-realistic images from sketches. However, GANs have some limitations, including instability during training, a lack of firm theoretical grounding, and the possibility for them to generate highly realistic fake images or videos, which may present ethical concerns.
Variational Autoencoders (VAEs)
Variational Autoencoders (VAEs), on the other hand, are a type of generative model that allows complex distributions to be encoded and decoded. They are crafted to compress data into a lower-dimensional space (encoding) and then reconstruct the original input from this lower-dimensional representation (decoding).
VAEs can generate new data that is similar to the input data, making them helpful in applications such as image generation, anomaly detection, and generating music. The main advantage of VAEs is that they provide a more robust mathematical framework and are easier to train compared to GANs. However, one limitation of VAEs is that the images or data they generate are usually blurrier or less sharp than those generated by GANs.
Understanding the Significance of Generative AI
Generative AI is becoming a rapidly influential factor across various sectors as it carries the potential to instigate a paradigm shift. Some of the most highlighted examples can be seen within the entertainment industry where it’s used to generate original music, design digital artwork, or even script movies. In the healthcare sector, generative AI’s capability to produce synthetic data is advancing research without endangering patient privacy. Furthermore, its use in developing simulations and scenario planning across a range of industries is enhancing decision-making processes.
Despite the tremendous upside benefits, it’s also vital to acknowledge potential pitfalls and ethical concerns tied to generative AI. This includes the disturbing trend of deepfakes and misuse of synthetic data. As such, fostering a comprehensive understanding of these AI models and their implications becomes imperative. This includes not only understanding how these models function but also knowing their strengths and limitations to maximize their utility and minimize potential threats.
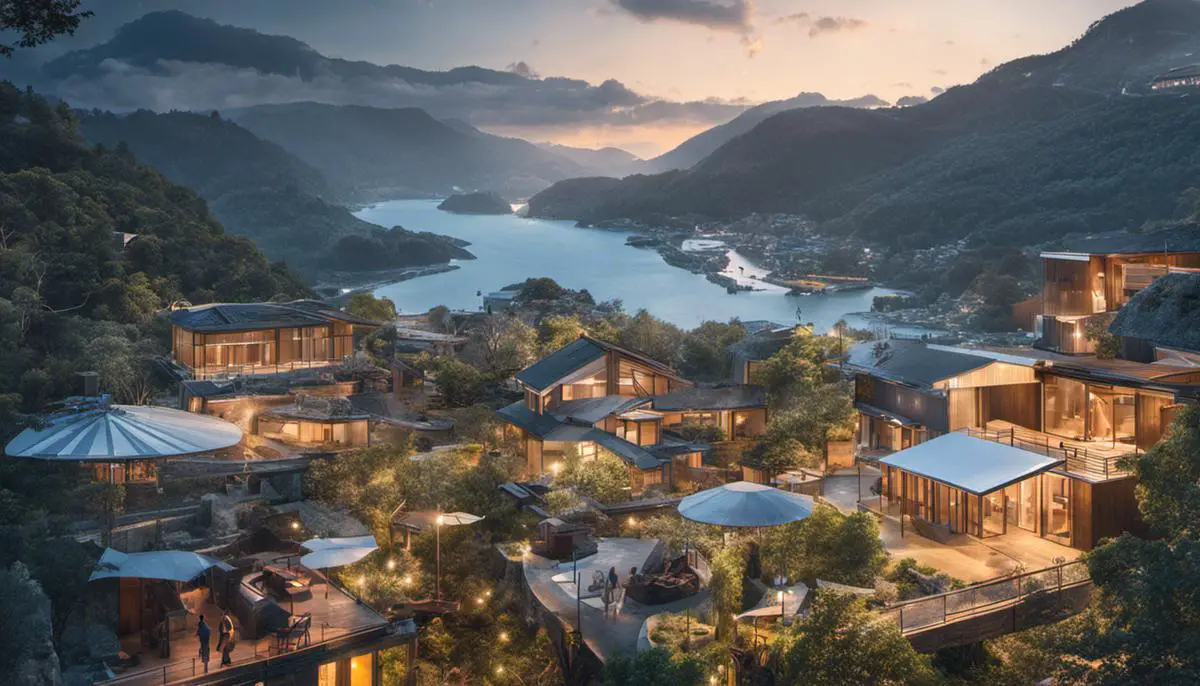
Real-World Applications and Examples of Generative AI
Exploring the Role of Generative AI in Art and Entertainment
The noticeable progress of Generative AI is especially highlighted in the world of art and entertainment. Applications like DeepArt and DeepDream effectively use convolutional neural networks, a subset of deep learning models, to transform images into intricate works of art. In the realm of music, an example of generative AI is AIVA (Artificial Intelligence Virtual Artist), which produces novel music compositions by leveraging deep learning networks.
In addition, the film industry is deploying generative AI to create lifelike visual effects and animations. There’s also the breakthrough use of AI in scripting like in the case of the short film ‘Sunspring’, which was crafted by an AI screenplay writer.
Generative AI in Business and Marketing
In the business realm, an excellent example of generative AI is in content creation and marketing strategies. Companies are using AI technologies to generate insightful marketing copy and personalised email correspondence that can better engage audiences. Platforms such as Jasper, Copy.ai, and Phrasee use natural language processing and machine learning to write unique and contextually appropriate content.
In addition, generative AI is used in creating customer profiles for targeted marketing. Platforms like Heliograf and Wordsmith are capable of writing news articles, financial reports, and sports news with increased speed and efficiency.
Generative AI in Design and Architecture
Generative AI has also found its application in design and architecture, transforming the way spaces are created and experienced. For instance, Autodesk’s Dreamcatcher system uses generative AI to go beyond traditional design software, creating thousands of design options based on given constraints and goals.
Also, companies are exploring AI’s potential in fashion design, where AI can generate innovative and unique clothing designs. Google’s Project Muze and designs by the fashion brand Ivy Revel are prime examples of AI-powered fashion design.
Generative AI in Healthcare and Medicine
In the field of healthcare, generative AI has shown promise in drug discovery and design. Atomwise’s AI technology has been used to predict which molecules may have a therapeutic effect on the Ebolavirus, potentially cutting down years of physical testing.
Moreover, the development of GANs – Generative Adversarial Networks, has opened up new possibilities like generating synthetic medical imaging data for training AI models, which can help meet the demand for high volumes of medical data while addressing privacy concerns.
Generative AI is a transformative technology that is gaining traction in numerous fields, from creative arts and hard sciences to business. This innovative AI approach is not just enhancing our understanding and interaction with our environments but is also becoming a vital tool in fueling creativity and efficiencies across multiple sectors.
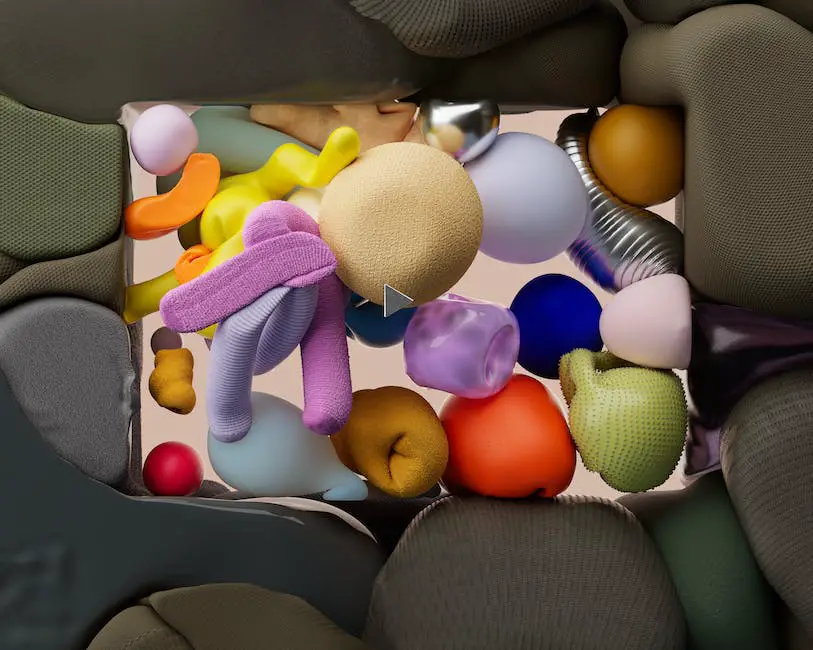
Future Perspectives and Ethical Considerations of Generative AI
Exploring the Future of Generative AI
With its ability to create high-quality content, designs, and solutions, generative AI holds significant potential for businesses and industries alike. This dynamism presents the opportunity to break new ground in various sectors. Think of it generating authentic designs in fields like fashion and interior design, drafting unique written material for journalism or advertising, and even concocting new music compositions.
Within the healthcare domain, imagine the positive impact of generative AI in devising new drugs and aiding the discovery of avant-garde therapies for a range of diseases. It can also create 3D models of molecules, simplifying the process of understanding their structure and attributes.
In education, the potential for generative AI is significant. Consider a world where virtual tutors adapt to a student’s learning style and preferences, generate answers to questions, and facilitate the learning process in a personalized way. With AI acting as personal companion for students, the overall learning experience could drastically improve.
Challenges of Generative AI
However, while generative AI provides promising possibilities, it isn’t without its challenges. For instance, it requires extensive computational power and massive data sets to function effectively. Amassing a high-quality, diverse, and representative dataset could prove challenging due to cost constraints, privacy concerns, or legal issues.
Another challenge is in the area of quality control. As AI-generated content or design increases in complexity, it can be difficult to ensure that the output always meets the required standards. There might be inaccuracies, inconsistencies, or ethical issues that arise from the generation process.
Ethical Considerations of Generative AI
The use of generative AI also raises some ethical considerations. For instance, there’s the potential risk for misuse of technology in generating deepfakes – the practice of superimposing existing images or video footage onto source images or videos using a form of artificial intelligence. Deepfakes can be used maliciously to create misleading video or audio recordings that portray people saying or doing things they never did. This can have serious implications for individuals’ privacy, consent, and rights to their own likeness.
There’s also the concern of job displacement. With generative AI becoming increasingly adept at creating high-quality content, the demand for human workers in fields like photography, music, journalism, and architecture could decline.
Equity in AI technology access is another ethical concern. Currently, the companies and countries with the financial means to invest heavily in AI research and development are the ones driving advances. Small companies and lower-income countries risk being left behind, creating an AI divide.
In summary, while generative AI offers exciting possibilities, it’s crucial to weigh its benefits against the potential limitations and ethical implications. Key considerations should involve transparency, responsibility, and equity in how AI tools and systems are developed and used, ensuring that they benefit rather than harm society. Technology developers, users, and policy-makers alike share the responsibility of ensuring a balanced approach towards the growth and use of generative AI.
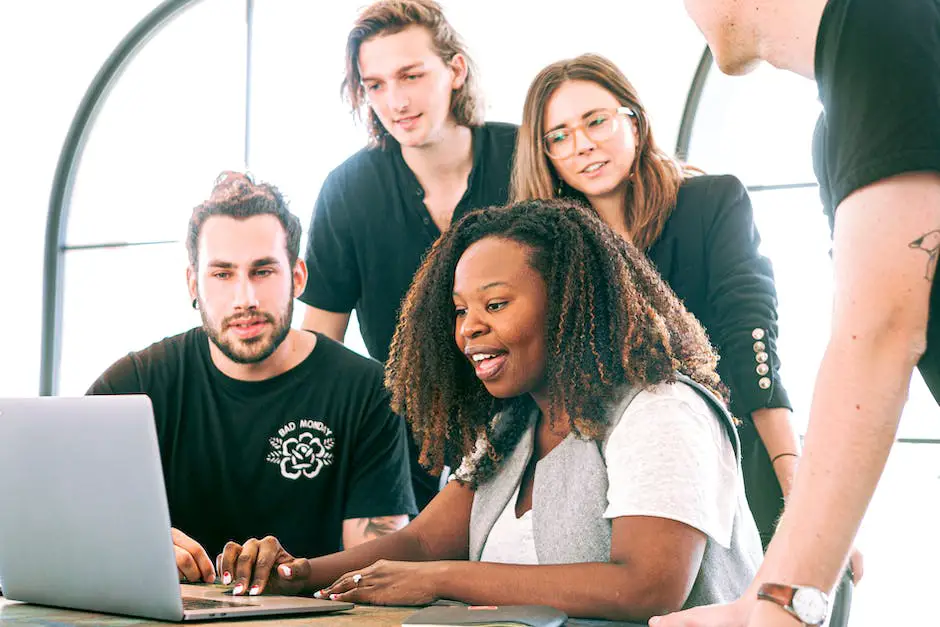
Generative AI, with all its complexity and potential, is not merely cutting-edge technology; it has started to become a significant tool that is reshaping various sectors including arts, business, entertainment, and more. Weaving intricate patterns within endless industries, this technology hints at an exciting future brimming with unprecedented possibilities.
However, as we step forward into this uncharted territory, it is equally crucial to be aware of the ethical implications that come into play. AI, if not managed carefully, has the potential to impact societal structures significantly. Hence, a balanced perspective, meticulous understanding, and cautious approach are indispensable as we venture further into this intriguing, promising, and complex world of generative AI.