Program Structure and Specializations
Master’s in Machine Learning programs in the USA typically include core courses, electives, practicum experiences, and specializations.
Core courses often cover:
- Introduction to Machine Learning
- Deep Learning Fundamentals and Advanced Techniques
- Probabilistic Graphical Models
- Convex Optimization
- Probability and Statistics
Programs emphasize practical application through practicum or capstone projects. For example, Drexel’s program concludes with a two-term capstone project.
Elective courses offer specialized knowledge in areas such as:
- Machine Learning with Large Datasets
- Natural Language Processing and Computer Vision
- Advanced Algorithms
Many institutions offer specializations or tracks, like:
- Applied Track: Focusing on practical applications in industry settings
- Computational Track: Exploring algorithm development and theoretical foundations
For instance, Drexel’s program provides Applied and Computational tracks. Carnegie Mellon’s electives cover topics like Generative AI, Machine Learning Ethics, and AI in Healthcare.
Hands-on experience through internships or co-op options is often available. Students at Duke engage in practical data science and machine learning projects.
Advanced courses may include:
- AI Governance
- Robustness in Shifting Environments
- Neural Networks for NLP
These programs aim to connect theoretical concepts with practical implementation, preparing students for the machine learning field.
Admission Requirements and Prerequisites
Typical prerequisites for Master’s programs in Machine Learning in the USA include:
- Undergraduate degree in a related field (computer science, engineering, mathematics, or data science)
- Minimum GPA of 3.0 on a 4.0 scale
- Foundational knowledge in calculus (up to Calculus II or III)
- Statistics coursework (including Probability and Mathematical Statistics)
- Programming experience (Python, Java, or C++)
Some programs recommend submitting Graduate Record Examination (GRE) scores, especially if the applicant’s GPA is below the required threshold.
Other application components include:
- Letters of recommendation (usually 2 or more)
- Statement of purpose
- Current resume
- Proof of English proficiency for international students (TOEFL or IELTS)
Some programs may request an introductory video or take-home projects to assess the applicant’s skills further.
Program Duration and Formats
Master’s in Machine Learning programs in the USA typically span one to two years. Different learning modes are available:
- In-person programs: Offer immersive experiences with direct interaction and access to on-campus resources.
- Online programs: Provide flexibility for students balancing professional commitments or preferring remote study.
- Hybrid programs: Combine online coursework with periodic on-campus sessions.
Traditional course-by-course curriculums follow a structured learning path. Modular certificate curriculums offer a more flexible approach, allowing students to build a customized education path.
The choice between these formats depends on individual learning preferences, career goals, and personal circumstances.
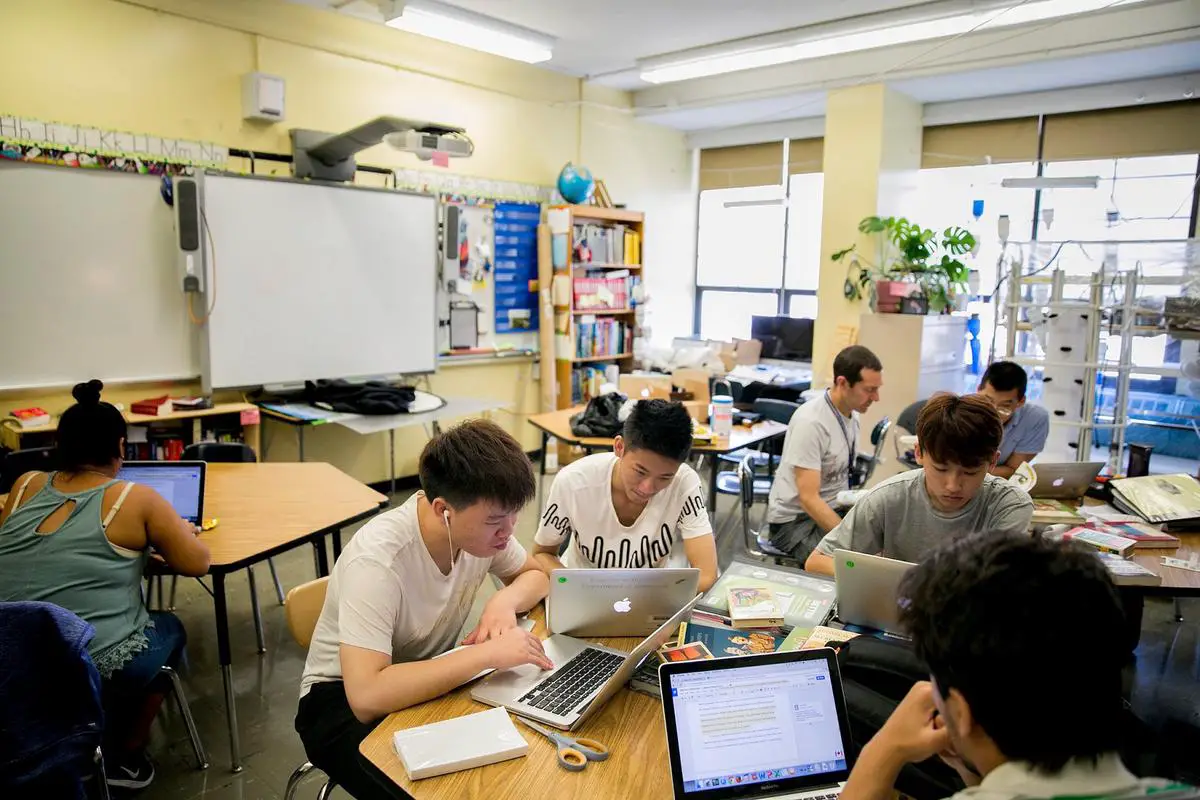
Financial Considerations and Scholarships
Tuition costs for Master’s in Machine Learning programs vary significantly. For example:
- Duke University: $99,734 – $113,892
- Johns Hopkins University: $52,700
- Purdue University: $28,000 (online)
Students should also consider associated costs such as materials, technology fees, and residency requirements for hybrid programs.
Financial aid options include:
- Institutional scholarships
- Merit-based scholarships
- Need-based grants
- Assistantships and work-study opportunities
External scholarships are available from organizations like the National Science Foundation (NSF) and tech companies such as Google and Microsoft.
Prospective students should research these options and contact financial aid offices at their chosen institutions to maximize their financial support.
Career Prospects and Industry Connections
Graduates with a Master’s in Machine Learning often secure roles in software, technology, finance, healthcare, and research. Common job titles include Machine Learning Engineer, Data Scientist, AI Engineer, and Research Scientist.
Starting salaries for Machine Learning Engineers and Data Scientists typically range from $100,000 to $130,000 per year.1
Many institutions integrate industry connections and practical experiences into their curricula:
- Drexel University offers co-op and internship programs
- Duke University includes a mandatory summer internship
- Stanford University has connections with top-tier tech companies
- Carnegie Mellon University maintains an extensive network of industry partners
Universities often provide career services, including resume workshops, mock interviews, job fairs, and recruiting events.
“These programs aim to equip students with knowledge and skills while providing opportunities for practical experience and industry networking.”
Special Features and Unique Aspects
Many Master’s in Machine Learning programs offer distinctive features that appeal to prospective students:
- Drexel University: Provides a co-op option for full-time, on-campus students, allowing them to gain real-world work experience. The program ends with a two-term capstone project and offers Applied and Computational tracks.
- University of Arizona: Uses a hybrid learning model, combining online learning in the first year with on-campus education in the second. This approach offers flexibility and cost savings while still providing an on-campus experience.
- Johns Hopkins University: Emphasizes flexibility through entirely online formats. Students have up to five years to complete ten courses, allowing them to balance professional and personal commitments.
- Northwestern University: Combines a traditional track with the MSAI+X program, integrating AI with the student’s original field of study. This interdisciplinary approach prepares students to apply AI principles across various sectors.
- University of Texas—Austin: Offers a modular certificate curriculum, allowing students to focus on specific aspects of AI and machine learning. This method aids in achieving incremental learning milestones and suits working professionals.
- University of Michigan-Dearborn: Provides flexible options of online, in-person, or hybrid learning, with concentration tracks such as Computer Vision and Machine Learning. Late afternoon and evening classes cater to working professionals.
- Carnegie Mellon University: Offers an extensive selection of elective courses and special topics in machine learning, frequently updated to include the latest advancements in the field.
These programs combine rigorous academic curricula with practical applications, flexible learning formats, and strong industry connections to prepare students for the dynamic field of machine learning and artificial intelligence.
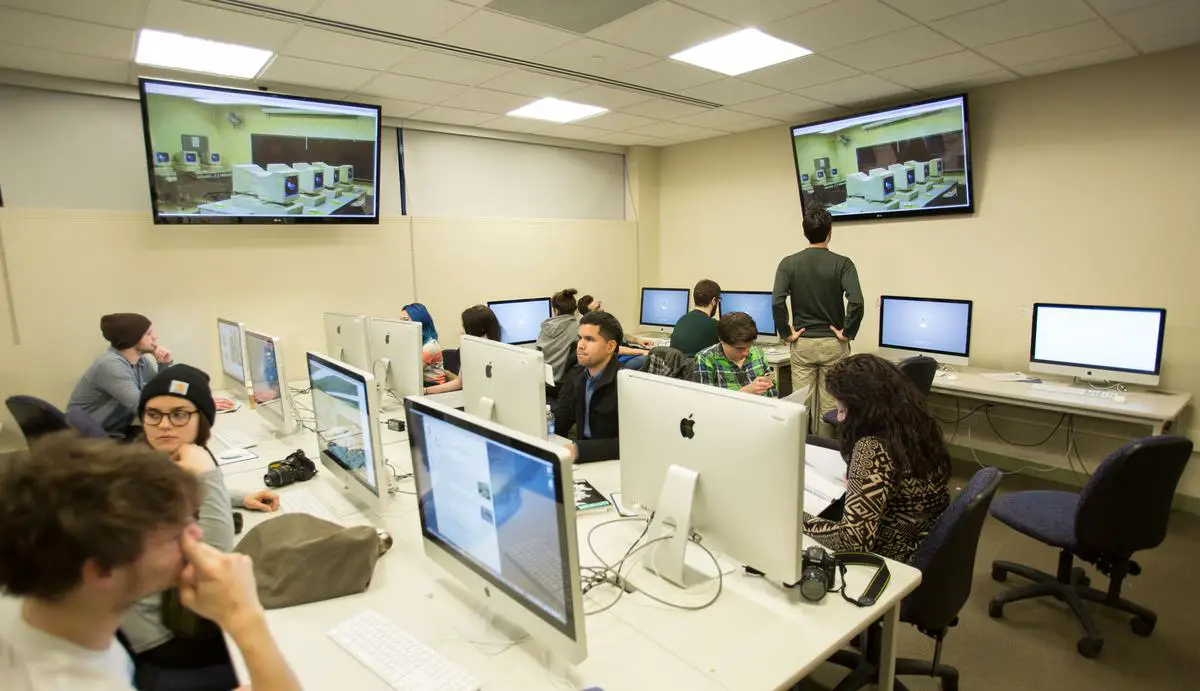
Graduating with a Master’s in Machine Learning opens doors to numerous high-paying and rapidly growing career opportunities. The combination of rigorous academic curriculum, practical application, and strong industry connections ensures that students are well-prepared to thrive in the fast-evolving landscape of AI and machine learning.
“The field of machine learning is not just about algorithms; it’s about reshaping the future of technology and society.” – Dr. Andrew Ng, Co-founder of Coursera and former head of Google Brain1
Indeed, the impact of machine learning extends far beyond the tech industry. From healthcare to finance, from environmental science to entertainment, machine learning graduates are poised to make significant contributions across diverse sectors.