At the crux of contemporary academic evaluation, Turnitin emerges as a seminal asset in preserving the integrity of scholarly work. Delving into its sophisticated detection capabilities, Turnitinโs robust algorithm scans the horizons of written assignments, tirelessly comparing them to an extensive repository of literature and online resources. This meticulous approach to safeguarding originality has become indispensable in the fight against plagiarism. However, as machine intelligence reaches new heights with creations like ChatGPT, the conversation shifts. We stand before the threshold of a new era, analyzing how such advanced technology weaves through the academic fabric, potentially leaving behind a trail undetectable by traditional methods.
Understanding Turnitinโs Detection Capabilities
Turnitinโs Battle Against AI-Generated Text: A Look Inside the Algorithm
In the swiftly advancing landscape of technology, artificial intelligence (AI) has undeniably clinched a vital position, particularly in text generation. But with great innovation arises the challenge of academic integrity. Hereโs where Turnitin steps in, brandishing its algorithm like a sword to safeguard originality. This software, a mainstay in educational institutions, has evolved to detect text generated by AI, a task thatโs far from trivial.
Breaking down the functionality of Turnitinโs algorithm reveals that itโs not just a one-trick pony. Instead, it operates on a multi-faceted approach, scrutinizing documents against a colossal database of academic papers, web pages, and publications. But AI-generated text is a different beast altogether, often slipping through cracks that would trap human-copied content.
To adapt, Turnitin employs a nuanced methodology. One core component is stylometry, the analysis of writing style. AI has a telltale signature, marked by certain patterns in sentence structure, word choice, and grammar. The algorithm dissects text characteristics that seem inhumanly uniform or deviate from typical student work. For example, AI might overuse certain transition phrases or exhibit a lack of idiomatic expressionsโa red flag for Turnitinโs watchful eyes.
Another tactic in Turnitinโs arsenal is the examination of semantic coherence and factual consistency. Tools empower the algorithm to determine whether content aligns with known information or derails into the nonsensical, a common pitfall when AI attempts to tackle complex topics without true understanding.
Moreover, metadata analysis also plays a pivotal role. Metadataโdata about dataโcan disclose a documentโs origins, and in the case of AI, anomalies such as rapid generation times or unusual creation processes raise immediate suspicion.
Yet, the algorithm doesnโt rest on these analyses alone. Machine learning amplifies Turnitinโs capabilities, digesting volumes of data to better discern the subtleties between human and machine-generated text. As AI text generators learn and adapt, so too does Turnitin, ensuring that its cutting-edge vigilance perseveres.
The outcome? Turnitin provides educators and institutions with a robust tool to uphold the sanctity of original work. The stakes are high in maintaining academic rigor, and Turnitinโs multi-pronged, tech-savvy strategy of leveraging stylometry, semantic analysis, metadata scrutiny, and machine learning is a testament to its ongoing commitment in the digital age.
AI-generated text may be an increasing concern, but itโs clear that Turnitin is not backing down from the challenge. As AI continues to mature, anticipate Turnitin to fiercely evolve alongside it, ensuring a fair playing field in the realm of education and beyond.
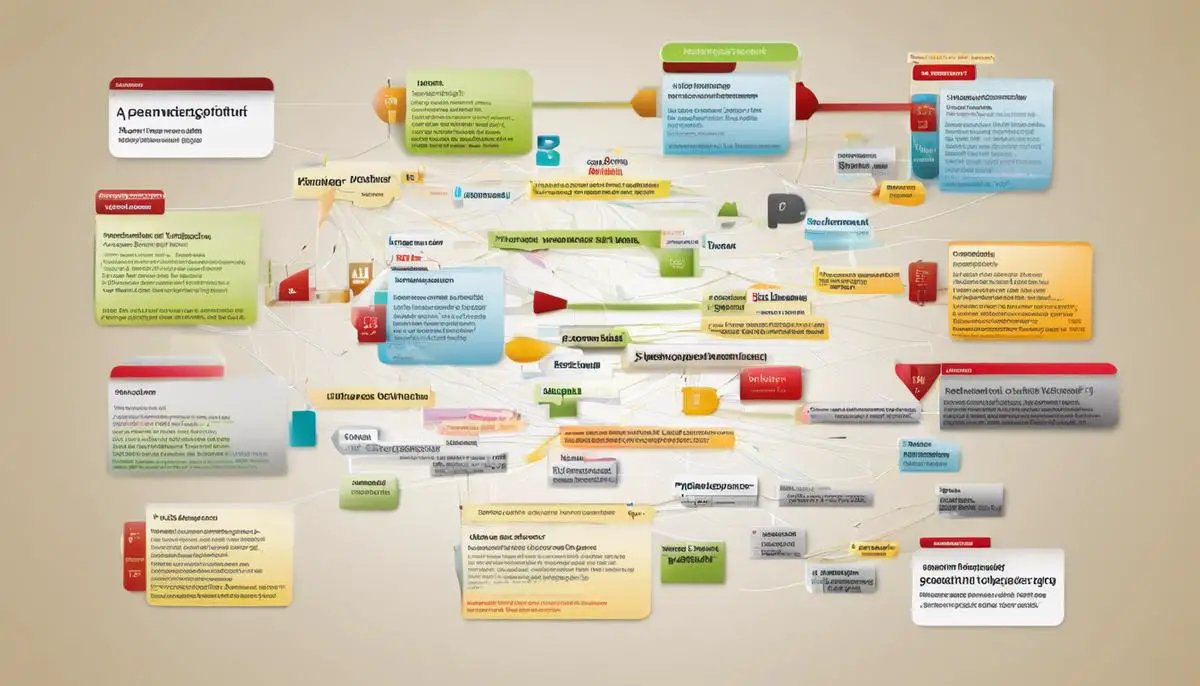
ChatGPTโs Footprint in Academic Writing
Beyond Turnitin: Tracing the Digital Fingerprints of AI Like ChatGPT in Text Creation
In the ever-expanding digital world, artificial intelligence has begun to leave its mark on the written word. AI systems, including prominent language models like ChatGPT, are now delivering sophisticated and human-like text. Yet, with the advancement of AI comes the necessity to discern the origin and authenticity of content, making it crucial to understand the unique patterns AI leaves behind in its writing.
AI-generated texts have telltale signs, intertwining sequences and nuances that, while invisible to the untrained eye, become evident upon closer examination. One such pattern is the AIโs penchant for repetitive structures. Unlike human writers, AI programs can employ reiterative syntactic configurations with consistent precision, often without the variability that a human writer would introduce.
Another clue lies in the realm of creativity โ or the subtle lack thereof. While AI can weave a narrative with apparent ease, its tales often lack the nuanced spark that is born from genuine human experience and emotion. Even though language models are armed with vast datasets of literary knowledge, the personal flair and original thought processes that characterize human writing are notably absent.
Additionally, AI is bereft of personal experiences, leading to a noticeable absence of first-person anecdotes or rich, personal details that typically color authentic human prose. The writing is scrubbed clean of the bias and uniqueness that each individual naturally imparts in their work.
While pattern recognition is fundamental, analyzing the linguistic cadence can also be enlightening. AI tends to follow a neutral, almost monotonous tone, steering clear of extreme language unless expressly programmed to mimic such styles. Its precision in language choice frequently leads to prose with grammatical flawlessness, though sometimes it runs into the uncanny valley of being almost too perfect, particularly considering human tendencies toward occasional slips and idiosyncratic expressions.
One methodical aspect of AI writing is its consistency in vocabulary and phraseology. AI algorithms may latch onto specific words or phrases, repeating them with a frequency that stands out upon scrutiny. The repetition isnโt the only factor; the depth and breadth of its vocabulary usage can seem conspicuously controlled and calculated, detouring from the random, creative nature of human linguistic expression.
The contextual understanding of AI is another hotspot for identifying its fingerprints. While AI can manage coherent dialogue and maintain topic relevance, it may fall short in subtly shifting between related topics in the way a human would. Transitions in AI-generated text may be smoother but also more mechanical, lacking the organic flow and sometimes messyโbut genuineโtangents of human thought.
In understanding these patterns, one must be wary of over-relying on them as definitive indicators. AI writing capabilities evolve continuously, and with each iteration, the distinctions become more subtle, more refined. Yet, for technology enthusiasts and the analytically minded, the thrill lies in the perpetual game of cat-and-mouse between AI text generators and the sleuths aiming to unearth the digital origins of content. Itโs this dynamism at the intersection of technology and language that fuels the ongoing innovation in fields like AI detection and creates a landscape where, despite the advancements, human touch remains distinguishable, at least for now.
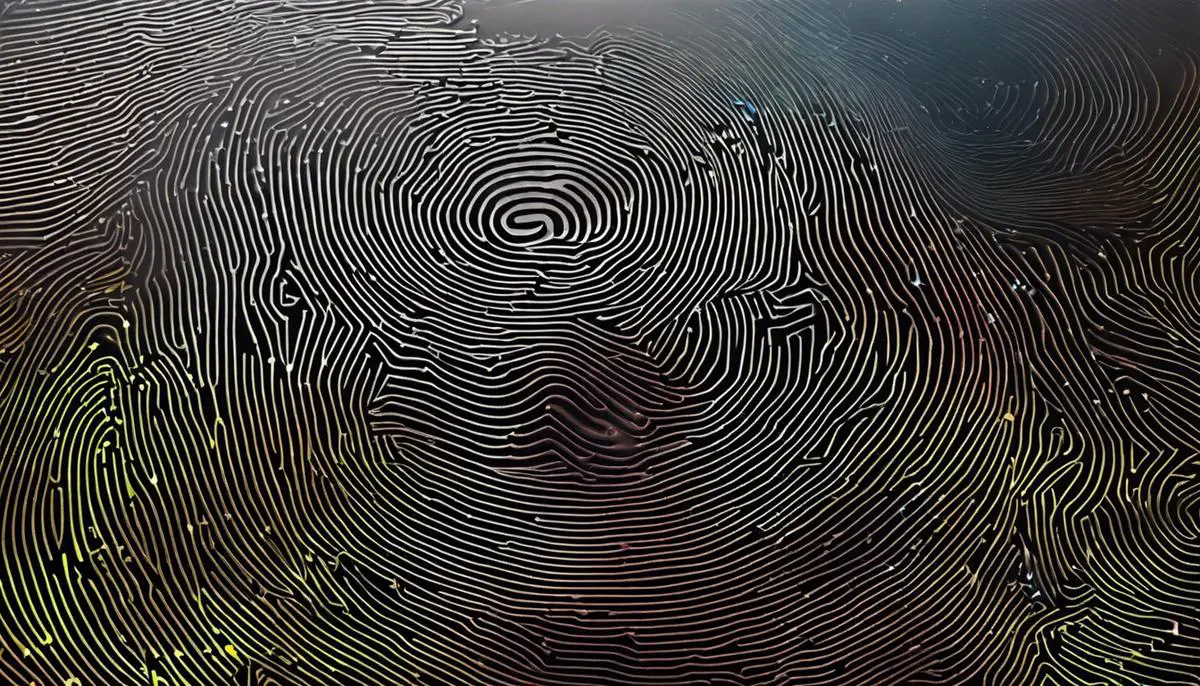
Advancements in Anti-Plagiarism Technology
Innovations Enhancing Turnitinโs Detection of ChatGPT Output
Educational institutions rely significantly on Turnitin to preserve the essence of scholarly work by discerning original student submissions from plagiarized ones. With the advent of sophisticated AI like ChatGPT, the arms race between cheating methods and academic integrity tools is heating up. Developers are ceaselessly crafting advanced technologies to enhance Turnitinโs detection capabilities, ensuring that the integrity of academic work remains unblemished by AI-assisted shortcuts.
Machine learning is a cornerstone of the innovation in detection methodologies. Algorithms are not static; they adapt and learn from the vast pools of data they analyze. Previous detection methods focused on matching text to a database of existing work, but the nuance of AI-written text demands a more intricate approach. Todayโs algorithms are increasingly skillful in pinpointing subtler facets of machine-generated content, like abnormal patterns in text flow and too-perfect grammar that typically lack the imperfections seen in human writing.
Neural network advancements are at the frontline of this campaign. These sophisticated systems can discern between human and AI-generated texts with impressive accuracy. By training on myriad examples of both, these neural networks develop a โsenseโ for the textโs origin, becoming increasingly proficient in signaling content that bears the fingerprints of algorithms like ChatGPT.
Another promising avenue is the integration of authorship authentication tools. These tools profile an authorโs unique writing style โ their syntactical preferences, common errors, and idiosyncratic choices โ and flag discrepancies that might indicate a section of content wasnโt penned by the student but generated by an AI.
Beyond syntax and style, emergent technologies are looking at conceptual consistency. AI-generated content often struggles with maintaining a consistent argument or point of view. Algorithms are being tuned to scrutinize the logical progression of ideas, watching for the telltale shifts that might suggest a text was assembled by a software lacking true understanding.
Embracing the intricacies of language and logic necessitates advancements in natural language processing (NLP). Turnitinโs arsenal now includes more advanced NLP tools that delve into the semantics or intended meaning behind a text, rather than just analyzing the surface text. By getting to the crux of meaning in written work, these systems are better equipped to root out inconsistencies emblematic of AI-generated content.
Combatting AI goes beyond the text itself, as metadata holds keys to authenticity. Innovations in metadata analysis contribute significantly to this battle. Since documents generated by ChatGPT carry distinct digital signatures, exploring a fileโs metadata can yield clues to its origin. For example, timestamp inconsistencies or unusual edit histories can suggest something is amiss.
Lastly, fostering collaboration between universities, tech companies, and AI developers ensures a proactive approach. Shared knowledge bases and open-source initiatives help fortify Turnitinโs database, enabling rapid updates to the platform when new AI writing patterns emerge.
In conclusion, maintaining academic standards in the age of AI-generated content is a challenge met by relentless innovation. With a fierce commitment to academic honesty, Turnitin and the broader educational technology community are constantly evolving, employing machine learning, neural networks, authorship profiling, advanced NLP, and metadata analysis to stay ahead. This unwavering determination not only safeguards originality in writing but upholds the value of human intellect and creativity in education.
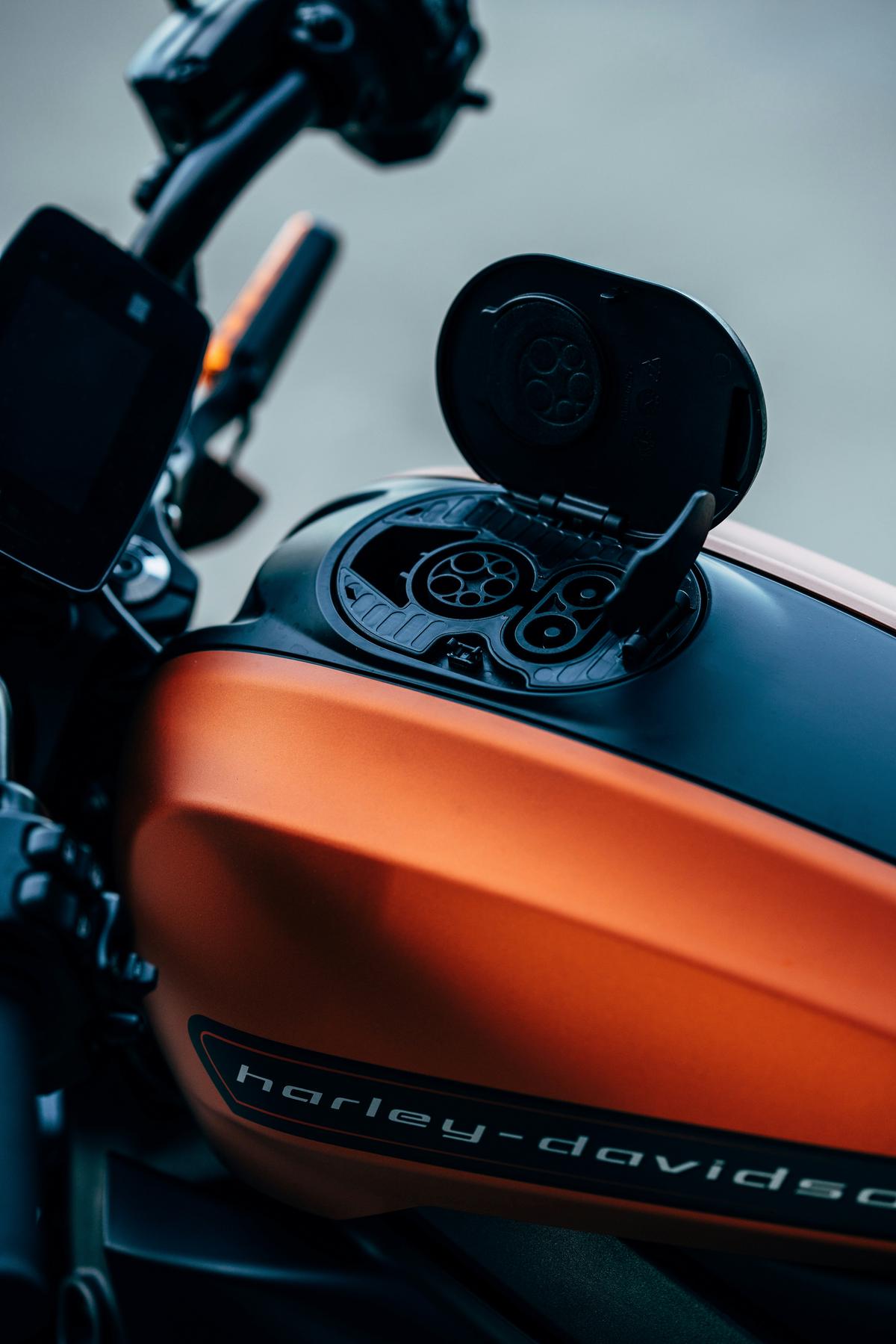
Photo by harleydavidson on Unsplash
Ethical Considerations and Academic Policies
The Intersection of AI and Academic Writing: A Critical Look at Integrity Policies
In the academic realm, integrity is a cornerstone that upholds the value of scholarly work. Yet, as artificial intelligence forges new frontiers in text generation, institutions grapple with unprecedented challenges. The very essence of originality is put to the test as AI-crafted essays and papers begin to permeate educational echelons.
Foremost, the distinction between human-produced and machine-generated content becomes murky. AI tools, with their advanced language models, are capable of churning out compositions that mimic the nuanced thought patterns of a human writer. Institutions now face a daunting task: adopting policies that recognize the finesse of AI while safeguarding against its potential for misuse.
Acknowledging this, academic bodies are swiftly revising policies to specifically address AI-authored submissions. Integrity guidelines, once mainly focused on preventing plagiarism among students, now expand their purview. The new policies must account for work which, while not plagiarized per se, lacks the personal intellectual engagement that true scholarship requires.
Central to new policy adaptations is clear articulation on AI use. Academic boards are designing frameworks that insist on transparency. Students and researchers must disclose any AI assistance, delineating the scope of its involvement. This promotes accountability and ensures that evaluators can clearly discern the origin and authenticity of intellectual efforts.
A key provision within these updated policies centers on delineating accepted versus unacceptable AI usage. In certain contexts, AI can serve as a beneficial tool, enhancing learner comprehension and providing adaptive feedback. Conversely, wholesale AI-generated texts masquerading as student work undermine educational outcomes. Clearly defined boundaries help maintain a healthy balance.
Enforcement poses another significant challenge. Striking a balance between fostering innovation and preventing misconduct is no small feat. Policies must not stifle the legitimate academic exploration of AI potentials, while still securing the ramparts against integrity breaches.
Privacy considerations are integral to this policy evolution. In the high-tech world of AI detection, educators and institutions must handle student data responsibly. Policies should underscore data protection and outline the measures in place to ensure that personal information isnโt misused or compromised in integrity-verification processes.
Educators, too, are called to adapt. They must hone their abilities to discern AIโs influence on student work. Professional development focused on recognizing the subtle characteristics of AI-generated text is increasingly vital. This empowers faculty to uphold academic standards through a more informed perspective.
In conclusion, the rise of AI in academic writing necessitates a dynamic response regarding policies on academic integrity. Institutions must craft astute, flexible guidelines that address the full spectrum of concerns. They also need to foster an environment that respects innovation and learning advancements, while not compromising scholarly principles. In an era of rapid technological evolution, itโs clear that both vigilance and adaptability are crucial in upholding academic excellence.
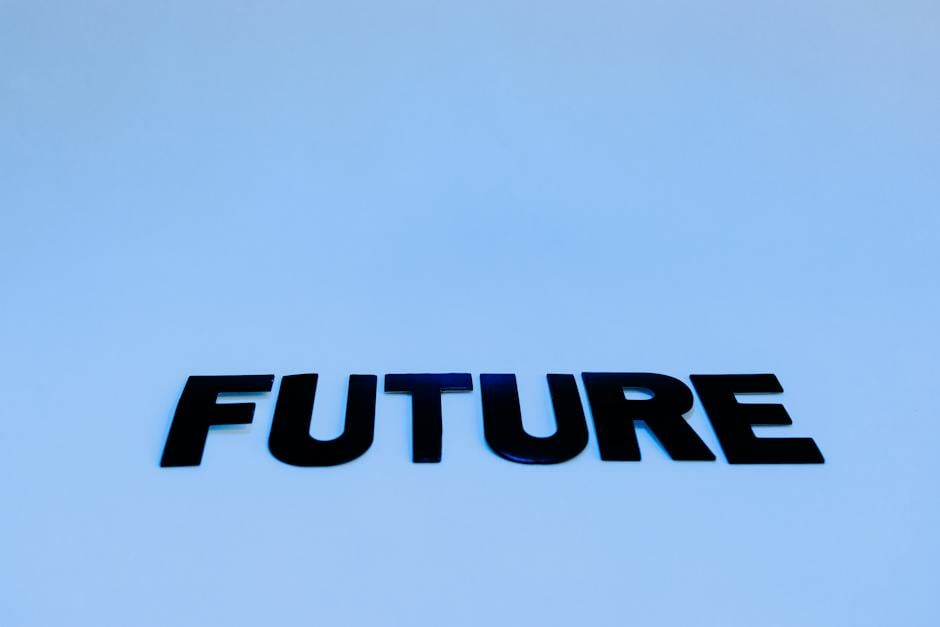
The landscape of academic writing is undergoing a transformation as artificial intelligence stakes its claim in the realm of content creation. With the advent of sophisticated tools like ChatGPT, educators and technologists alike are called upon to redefine the parameters of originality and authorship. It is an era where ethical discernment and technological innovation must coalesce to uphold the sanctity of academic work. The path forward demands a vigilant and adaptive approach, ensuring that our pursuit of knowledge remains enshrined in authenticity and that the value of human intellect is never shadowed by the capabilities of its artificial counterparts.